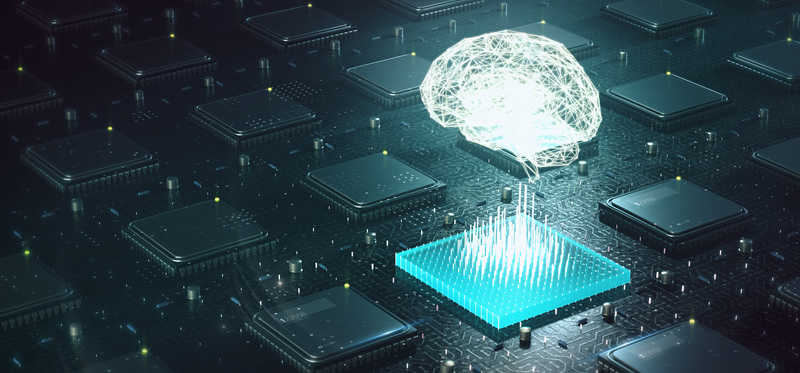
Frederick Vallaeys presented a session called “Will the Terminator Take My Job or Will I Run an Army of Robots?” at SMX Munich.
SMX Munich has become one of the most significant search marketing conferences on the planet and is visited by delegates from all over Europe.
So, a fitting place for Frederick Vallaeys, once described as the “most influential PPC expert in the world”, to make an appearance, leading a session with the terrifyingly scary title: “Will the Terminator Take My Job or Will I Run an Army of Robots?”. After Rand Fishkin’s keynote called “The Four Horsemen of the Apocalypse”, it was beginning to seem like a Hammer horror movie rather than a search conference.
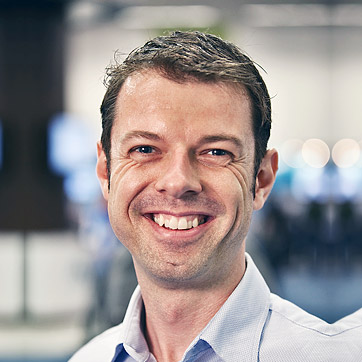
Frederick certainly has form in the PPC field. A native of Belgium who moved to Silicon Valley as a child, he worked for Google on the AdWords system for over seven years before leaving to form his own agency and the later a SaaS product called Optmyzr, that provides tools to help people be more efficient and achieve better results with what’s now called Google Ads.
So, back to robots. Actually, Frederick’s presentation was divided into two halves – the amazing things that AI and machine learning – i.e. robots – can do – and scripts you can use on Google Ads. I’m not sure that many people would think of scripts and AI in the same breath and almost certainly not robots. Scripts seem to make things seem ordinary and manageable whilst robots are something alien and potentially dangerous. (Mind you, scripts can be pretty deadly too – but not in quite the same way!)
He had some nice stories and some great lines from the blue-sky world of AI. Yes, you’ve probably heard that Deep Blue beat chess grandmaster Garry Kasparov, but did you know that it achieved that by preparing to beat Kasparov, rather than learning outstanding chess moves? It prepared for the opponent it knew it was getting, rather than all comers.
And then there’s the famous AlphaGo story, where for USD 250 million (which seems an awfully expensive app to get from the app store), software was built that could win against players in the game of Go. But Frederick’s best line was telling us that there are more possible permutations of moves in Go than there are atoms in the entire universe. I guess the price tag is small in view of someone having to do that count. Go figure!
Frederick also highlighted that humans can relatively easily outsmart predictable automations. Take for instance, self-driving cars. He tells the story of seeing a self-driving car heading towards him as he wanted to turn left and, knowing the self-driven car would break or swerve to avoid him, he turned directly across the oncoming vehicle in total safety. Bet he didn’t tell his mother that story, but what it shows is that he – the human – took advantage of what he knew the car’s computer would do in response to his move. (That’s actually been one of my points: when everyone is using the same core machine learning, the advantage will become to do something else than the predictable.)
Apparently, Google has found with self-driving cars that it needs to be a little bit pushier and more aggressive, if its cars are actually to get into traffic flows and not be found sitting at junctions with skeletons of former passengers who simply didn’t make it out as the world went by.
When PayPal needed to significantly improve its handling of fraud, it turned to machine learning to speed up the process and capture more instances of crime and that worked, to a degree. But it actually found that the best solution was to have humans overseeing a selection of potential fraud cases chosen by machine learning programs.
Jumping now to machine learning and Google and those scripts, Frederick highlighted a recent Google statement which said: “Google Ads is advertising that works for everyone.” This might seem like a relatively innocuous statement but, when you think about it, it’s really not. Google’s ad platform is definitively not straightforward and in fact is pretty darn complicated. It cannot be described as a tool that is suitable for “everyone” and nor does everyone get success from it. So, the meaning of Google’s statement must be increasing simplicity for the user. Increasing simplicity implies a lot more automation and more automation means a much greater use of AI.
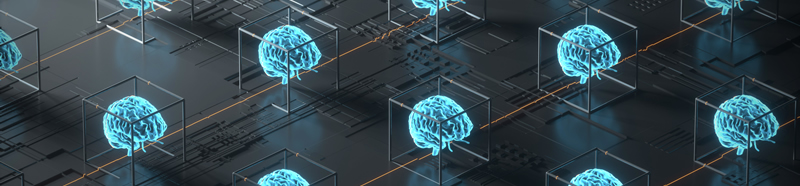
A recent Google innovation was interesting by way of giving an example of how Google is thinking – namely the arrival of “close variants”. Close variants is a good example of how Google is implementing machine learning techniques to deliver on making the system simpler to use for “everybody”, but in some ways, it is more difficult to control.
Essentially, in the early days, the “exact match” setting meant exactly what it said on the tin – but then in 2014 misspellings and plurals were added to make it exact-whether-users-can-spell-or-not match. Then, in 2017, word order became more or less irrelevant and function words such as conjunctions were included. So now we’d gone from exact match to exact-with-misspellings-plurals-upside-down-and-left-to-right-and-conjunctions-thrown-in match. Thank goodness for robots!
But there’s more – at the end of last year, Google added variations that have the same meaning or synonyms, giving us no-one-could-call-that-exact-anymore-apart-from-Google match. Makes you wonder if “broad match” isn’t actually narrower.
The name of the game was to try and reflect the intent of the user or their interest rather than expecting them to phrase the keyword exactly as the advertiser expected and Google is using machine learning to achieve this. (In the agency world, we all know that advertisers often have an idea about how their customers will search that doesn’t quite reflect the reality). Of course, agencies and advertisers will tend to fix any matches that are, shall we say, wide of the mark.
Frederick suggests we use the Levenshtein distance to solve the challenge of close variants. I know right, you knew that already. Everyone remembers Levenshtein – (who?)
Vladimir Levenshtein was a Soviet mathematician who created a concept called the “Levenshtein distance.” No, this isn’t how far you can leap after three vodkas, this is linguistics we’re talking. Basically, Levenshtein worked on error correction methods between words. If we take a target word (keyword) and compare another (think search query), we give points to determine how far apart they are, so a point for each character of difference, a point for each character deleted and a point for each letter added. The result is a score which measures the distance between the keyword and the search query which Google considers an exact match (except remember that means they have to use the same alphabet).
The Levenshtein distance can be incorporated into Google scripts which enable you to turn extravagantly selected wild matches – which score high on Levenshtein – into negatives. The funny thing is – what you’re actually doing is automatically turning off, to a large degree, Google’s own automation. All that effort – makes you think…
At this point, Frederick highlighted the irony of Google’s “smart bidding”. He said: “Google adds ‘smart’ to everything to make it sound better, even if it’s not so smart”. Smart bidding is powerful in the sense that bids are changed in the auction itself. The downside is that, like so much automation, there are significant gaps in what it understands. A strong example, for instance, is seasonality.
Seasonality is not fully accounted for in Google’s “smart” algorithms. You may need to act to vary, for instance, a CPA (cost-per-action) for areas such as weather or seasonality – for example, the algorithm will never accommodate a major event which means a change in the performance of the campaign.
One key takeaway from Frederick was that Google’s quality score has become much more important in the last year – something advertisers should take account of.
And one final point that my head is still coping with: testing A-A splits instead of A-B splits. In other words, you test the same ad against – yes, you’ve guessed it, the same ad. The difference is the context of the ads, as an ad in one ad group has its performance influenced by the history of that group. Put that ad somewhere else – the same ad – and its performance may be very different.
So, to sum up, the robots are coming but they don’t look like robots – in fact they look like speedy tricks to help the user do things more quickly – and they won’t make the tea for you (although those robots are coming too). Meanwhile, a key point of Frederick’s presentation was that machine learning needs humans and that humans + machine learning = best performance. Machine learning, AI or robots on their own, means getting stuck at the junctions like the self-driving car.

Andy Atkins-Kruger

Latest posts by Andy Atkins-Kruger (see all)
- Launching our new concept – Webcertain In-house! - July 26, 2019
- Yes, the robots are here and they’re running Google Ads! - April 10, 2019
- Be prepared: A personal message from Webcertain’s CEO - May 15, 2018